
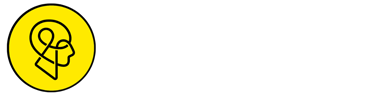
Exploring the frontiers of GenAI with a special focus on Infra & LLMs
Micro-summit series
March 27th
In-person & Virtual
Free to attend
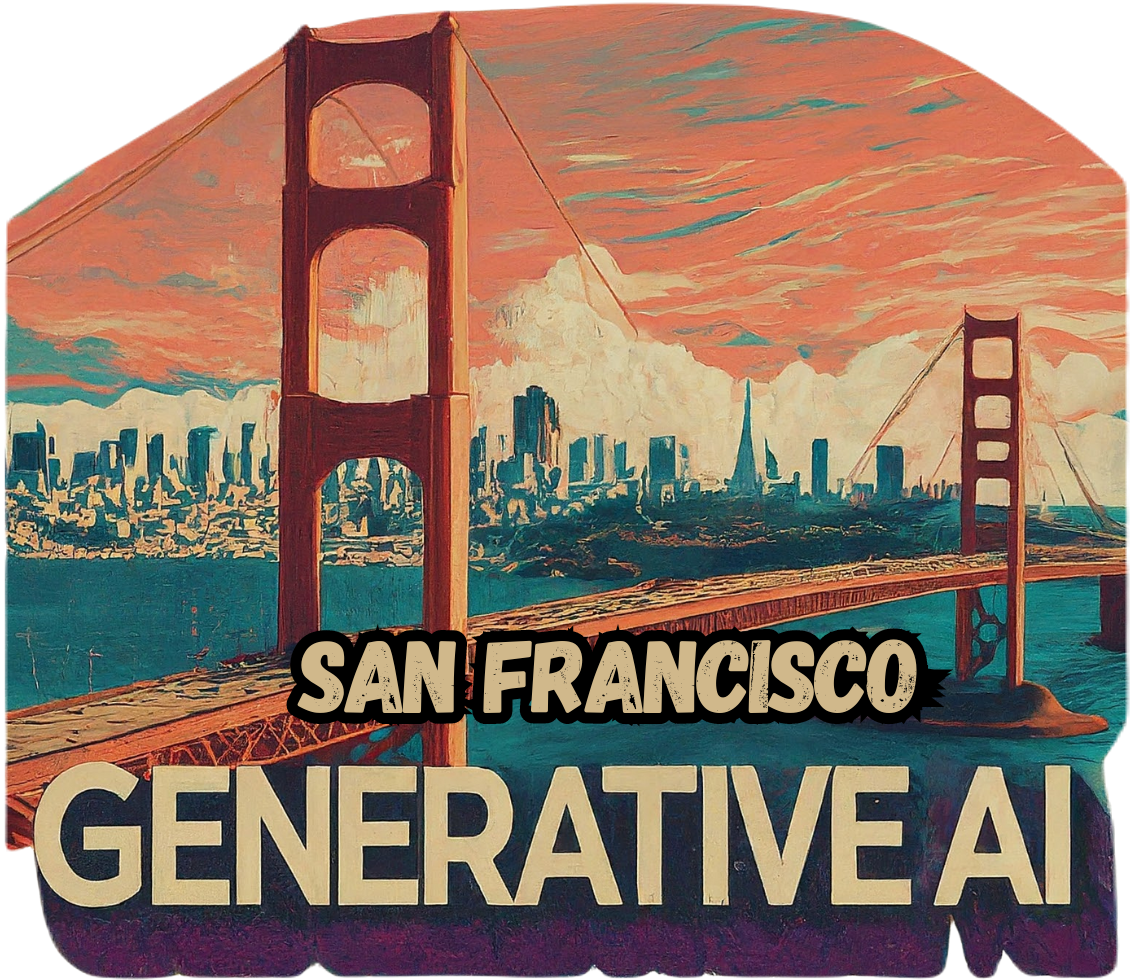
Join us for the San Francisco in-person & Virtual summit dedicated exclusively to GenAI Infra & LLMs.
- 2 Talks
- 4 Workshops
- Talks for beginners/intermediate & advanced
Understanding of the best practices, methodologies, principles and lessons around deploying Machine Learning models into production environments.
Speakers
Workshops
Tickets
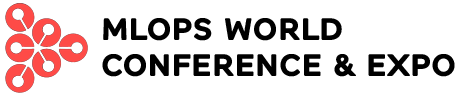
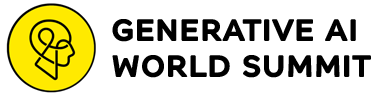
Micro-summit on GenAI
In-person & Virtual
Subject to minor changes.
Agenda
March 27th 2024
10:00 AM
PST
10:00 AM PST
Registration
10:15 AM
-
10:45 AM
10:15 AM - 10:45 AM
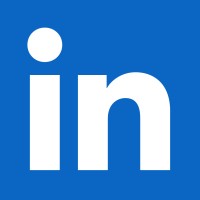
"MLOps at LinkedIn"
Dre Olgiati, Distinguished Engineer
LinkedIn
10:50 AM
-
12:20 PM
10:50 AM - 12:20 PM
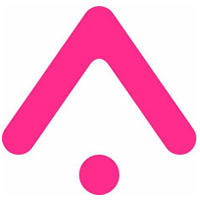
"Tracing and Evaluating a LlamaIndex Application"
Francisco Castillo, Software Engineer/Data Scientist
Arize AI
12:55 PM
-
1:55 PM
12:55 PM - 1:55 PM
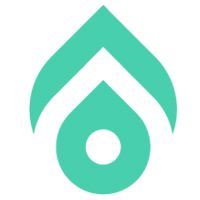
"Building A RAG Chatbot from Scratch with Minimum Hallucinations"
Niv Hertz, Director of AI
Aporia
2:00 PM
-
2:30 PM
2:00 PM - 2:30 PM
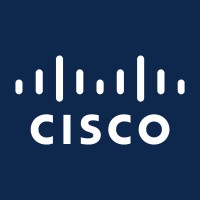
"Building Secure and Trustworthy Applications with Generative AI"
Kumaran Ponnambalam, Principal Engineer - AI
Outshift by Cisco
2:35 PM
-
4:05 PM
2:35 PM - 4:05 PM
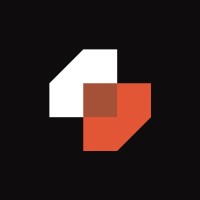
"Fine-Tune Your Own LLM to Rival GPT-4"
Alex Sherstinsky, Developer Relations Engineer
Arnav Garg, Machine Learning Engineer
Abhay Malik, Product Manager
Predibase
4:10 PM
-
5:40 PM
4:10 PM - 5:40 PM
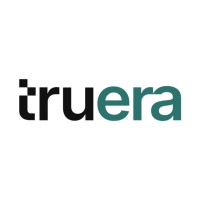
"Evaluating and Monitoring LLM Applications"
Anupam Datta, Co-Founder, President & Chief Scientist
Daniel Huang, Senior Software Engineer
TruEra