
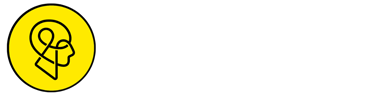
Exploring the frontiers of GenAI with a special focus on Infra & LLMs
Micro-summit series
April 15th
In-person & Virtual
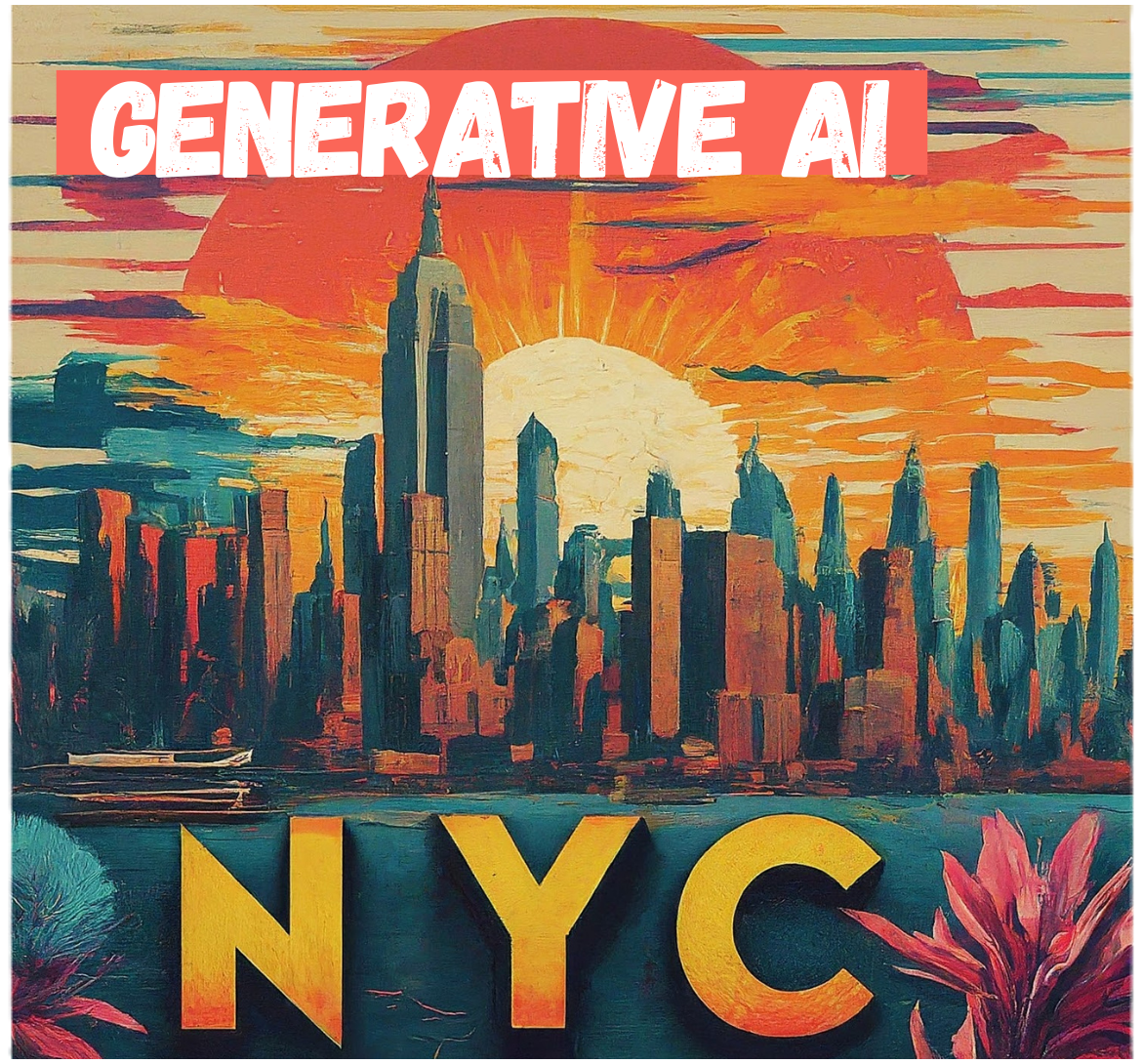
Join us for the New York City in-person & Virtual summit dedicated exclusively to GenAI Infra & LLMs.
The micro-summit includes:
- 3 hand-on workshops
- Post-summit networking
Understanding of the best practices, methodologies, principles and lessons around deploying Machine Learning models into production environments.
Tickets
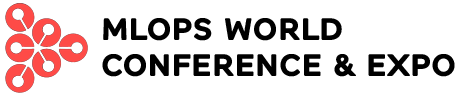
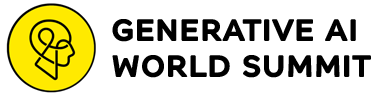
NYC Micro-summit on GenAI
In-person & Virtual
Subject to minor changes.
Agenda
April 15th, 2024
9:45 AM
EDT
9:45 AM EDT
Registration
10:05 AM
-
11:35 AM
10:05 AM - 11:35 AM
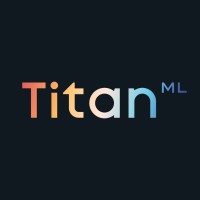
"Orchestrating AI Models for Powerful Applications"
Rod Rivera, Growth Lead
TitanML
11:40 AM
-
1:10 PM
11:40 AM - 1:10 PM
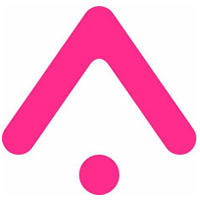
"Tracing and Evaluating a LlamaIndex Application"
Aman Khan, Group Product Manager
Arize AI
1:15 AM
-
2:45 PM
11:40 AM - 1:10 PM
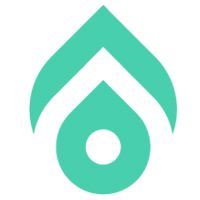
"Building A RAG Chatbot from Scratch
with Minimum Hallucinations"
Niv Hertz, Director of AI
Aporia
Join Our Community
Our goal is to provide an open, inclusive community of ML practitioners who can share projects, best practices and case studies. Join our open group, meet our community and share your work with practitioners from around the world.
Join us here and learn more: